Project Summary
This project aims to develop AI technology and software to provide actionable information for larger logistics operators to support their transition to cargo bike logistics.
Project Achievements
Activities A user interface was designed as a proposal for a complete user-facing application. The user interface allows operators to get an overview of their costs and revenue, identifies the optimal vehicle and depot investment strategy, and provides tools to further reduce costs and tweak their revenue model. The core simulation and optimisation technology was developedas an API allowing operators to integratethe tool into their own technology stack.
Conclusions
Our simulation engine provides a quick and inexpensive way for logistics operators to understand how they can reduce costs and carbon emissions by replacing fleet vehicles with electric cargo bikes and vans. Our platform accurately quantifies the speed and efficiency gains cargo bikes will provide operators by analysing their historical operationaldata. The wider impact is faster adoption of low-emission, low-congestion delivery vehicles to make our cities more liveable.
Next Steps
Algorithm Refinement We are currently exploring the use of advanced machine learning methods such as embeddings (compressing all the relevant urban featuresto a small vector space) to have models that can generalise to multiple cities and urban contexts. Commercial Partnership We are exploring a possibility of entering a commercial partnership with one of the mid-sized cargo bike operators for the further product development.
Featured In
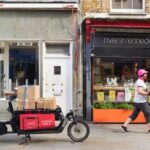
Article / Gearing up for a push on last mile eco-logistics
Climate technology firm Kale AI is on a mission to accelerate the growth of sustainable…