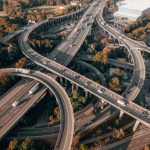
Working with our Data Science fellow Annamaria, who is a senior Data Engineer in Camden’s Data and Analytics team, this fellowship project focused on combining human centred design and data science guidance from the Catapult to explore how data science might be used to support residents at risk of rental arrears. The original aim of the project was to focus on reducing the number of people falling into rent arrears, by predicting which residents were most at risk of this so that the council could offer proactive support.
Working with the council, we took our first steps into using rent and data science to explore a number of areas. Our first step was to look at where people had aimed to do similar things before. We found some previous examples, including one from Hackney council; these examples were all similarly prediction-focused, but mostly because the organisation already had an intervention they used to “pull someone back” from being at a certain risk (for example, a nudge email reminding them to pay their rent), and wanted to better inform the time at which it was deployed.
We then assembled a stakeholder group within Camden’s housing team to understand if they were in a similar position, and more generally explore how they worked and what they would find useful.
We found they had no specific “let’s get this person out of arrears” interventions, and spoke about using a more holistic approach, as their housing officers work closely with tenants and engage with them not just about rent. The stakeholders discussed how someone who falls into rent arrears is likely to have other (often more urgent) issues at the root, and their interventions were usually more focused on how to deal with those root causes (for example, having lost a permanent job and taken a zero-hour contract, or having recently become a carer for a family member), rather than the specific arrears issue.
The real challenge for housing officers was working out what issue to deal with first, and what intervention to apply to it.
We ran a session of doteveryone’s consequence scanning – which we have found to be a helpful way of carefully considering how we push towards positive consequences with data work, particularly in complex areas that affect vulnerable people. We explored the potential unintended consequences of the work: for example any predictive analysis proliferating bias or being used to justify staff resource reduction. We wanted to be transparent about these with the stakeholder group and get their thoughts, understand and explore other concerns from their perspectives, and make sure the data science responded to any insights through methodology choices and scope refinement.
From our research both into previous projects and with the stakeholder group, we saw there was a need to focus on using data science to understand how significant different risk factors are for tenants falling into rent arrears rather than for prioritising who was most at risk of falling into arrears.
The ideal outcome would enable housing officers and policymakers to better prioritise interventions to deal with the root causes of tenants falling into rent arrears; and in the future, could be incorporated into a wider system to prioritise interventions based on multiple aims – not just to reduce the risk of rent arrears, but also to reduce the risk of a tenant developing stress-related health problems, for example.
The change of focus reflected the change in Camden’s strategy for managing rent arrears following the launch of the new Landlord service in June 2019. User research revealed a number of factors that play a key role in the risk of people falling into arrears. These included widely accepted factors such as property condition and household composition, and factors that refer to an individual tenant’s situation, such as being employed on a zero-hour contract basis, being unemployed and having particular vulnerabilities (for example problems with substance abuse or disabilities). Due to the sensitive nature of data in these areas a careful approach to information governance and ethics was critical. One helpful structure for ensuring appropriate and compliant use of data is a Data Protection Impact Assessment (DPIA). The DPIA involves explicitly stating the purposes of data processing and how it would benefit both Camden and the tenants, appropriate aggregation/anonymization techniques, and referral to specific legislation providing the legal basis for using such data. The consequence scanning and change in focus helped the Fellowship team reach a plan for analysis that used data carefully and appropriately, carefully justifying its use. In particular, a focus on explaining rather than predicting steered us away from potentially negative uses, keeping the housing officer in a key decision making role with information helping them but not letting data take control of important support decisions.
Building on our user research, discovery of data that capture aspects of factors likely to increase the risk of arrears meant close collaboration with Camden’s data engineering team to explore Camden’s data infrastructure. Several data sources were identified. These included variables related to payment patterns (such as regularity of rent payments and time in arrears) as well as variables holding contextual information on the propensity of falling into arrears (such as receiving housing benefits and being on universal credit). Some datasets were inevitably easier to access than others. As information cuts across different departments in the local authority, relevant and important information for this project, such as housing officer assessments, resides in data systems not necessarily connected to the core database. A data culture where different departments communicate the available data sources along with the necessary metadata is key for discoverability, and unblocks successful data science projects. With this in mind, we set up some structure for Annamaria and the data team at Camden to use in a data provenance exercise leading to a data catalogue, which can be built on over time and help identify new data projects of potential value.
Incorporating domain knowledge into the data science workflow is usually critical for a project’s success. The user research phase of the project revealed a fundamental, and often overlooked, element of managing rent arrears: it is the housing officers that hold key information regarding an individual tenant’s situation. By maintaining frequent correspondence with the tenants, the observations of housing officers can inform the potential reasons behind arrears. These can be anything from lack of skills and knowledge leading to poor budgeting or prioritisation, to events such as sudden loss of employment or health issues. By transforming this information into meaningful features we hoped to feed into a data science model. Such features involve identifying patterns related to the progress of a tenant’s situation as assessed by the housing officer, as well as identifying key concepts related to the difficulties a tenant may be facing when managing their rent. The former can be approached within a sentiment analysis framework, while the later using topic modelling techniques.
Rapid is Camden’s case management system, which stores comments and narrative around a tenant’s situation as freetext. As each individual case evolves, the longitudinal nature of these data means the possibility of identification of switch points that can indicate changes that might result in risk of arrears. But the nature of this dataset presents challenges when trying to extract semantic or contextual information using natural language processing techniques. The domain specific nature of the text data (which includes jargon, abbreviations/specific codes etc.) prevents using off-the-shelve NLP algorithms for sentiment or text analysis – a more bespoke solution is required. This means direct input from the housing officers in the initial stages of the modelling process, either through annotating text with specific tags (e.g. job loss, 0-hour contract etc.), or through identifying the general sentiment for a number of Rapid entries.
Annamaria started inspecting these data for use with the aim our user research and consequence scanning had refined in mind: supporting housing officers to understand and prioritise risk factors than can result in tenant arrears. To enable Annamaria and Camden’s data team to continue working towards using council data to help housing officers support residents, we put together a plan based on our learnings from the fellowship project to enable best practice and appropriate application of data science at the Council.
Extend the user research with housing officers
Firstly, to understand better how Rapid is used to record information on visits or calls with tenants (for sentiment analysis).
Secondly, to understand their perspectives of the factors that lead someone to be at increased risk of going into arrears – what have they noticed are some factors which seem to contribute to people who fall into arrears frequently?
Thirdly, to understand the interventions they’ve used before to help someone deal with those risk factors. Some of these came out during the initial research, but it was important that we could map these against the risk factors – and understand which risk factors were in the remit of the council and which weren’t.
Extend research with tenants
We hadn’t been able to speak to any tenants as part of this short project, but it’s a crucial step to engage with tenants to really be human-centred in our approach. Not only to explore their experiences of rent arrears and what impacts their ability to pay rent, but also to look at their interaction with the council and housing officers to understand how support might be improved.
Incorporate that knowledge into a modelling approach
Our focus so far has been on problem understanding, data identification and refining scope to ensure appropriate application of data science to support housing officers and tenants, steering away from negative unintended consequences. The lessons learned so far can guide us in constructing and implementing a model.
Given the focus on understanding risk factors, the modelling approach should reflect the need for an explainable, flexible and transparent way of understanding the factors behind arrears.
The model should incorporate information at different levels (e.g. individual factors, population level socioeconomic factors, factors related to the location of the property with respect to the wider environment or other properties etc.).
The model should also be flexible enough to account for different levels of uncertainty associated with each variable.
These requirements make the use of hierarchical modelling frameworks appealing. At the bottom of the hierarchy, the observation model should reflect any modelling assumptions related to the process of being in arrears at a point in time (e.g. using Generalised Linear Models). Framing the problem through a Bayesian approach would allow for incorporating different levels of prior information in the model’s hierarchy. For example, the degree of belief in the influence of socioeconomic status of an individual household to rent arrears could be informed by the levels of deprivation in the area the property is located. Likewise, the effect of the location of a property with respect to arrears levels of households in the vicinity can be represented using a neighboring structure, allowing clustering effects to emerge. Iteration and reevaluation of outputs should be included in the pipeline and should be scrutinised by domain experts (such as housing officers).
Continuing consequence scanning
The doteveryone consequence scanning activity has been picked up by Camden on other data projects, and proved a really useful tool. To be useful, this needs to be iterated on and reviewed, with an eye on mitigating negative consequences and giving everyone involved in the project opportunities to raise any emerging unintended consequences they saw.
The combination of data science and human centred design support on this data science fellowship proved invaluable – we believe this combined expertise is particularly important in shaping opportunities for getting value out of data, especially on tricky council problems where ultimately the aim is to support residents making best use of council resources.