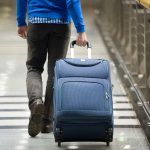
Security Technology Research and Innovation Grants (S-TRIG) – Weisi Guo, Cranfield University
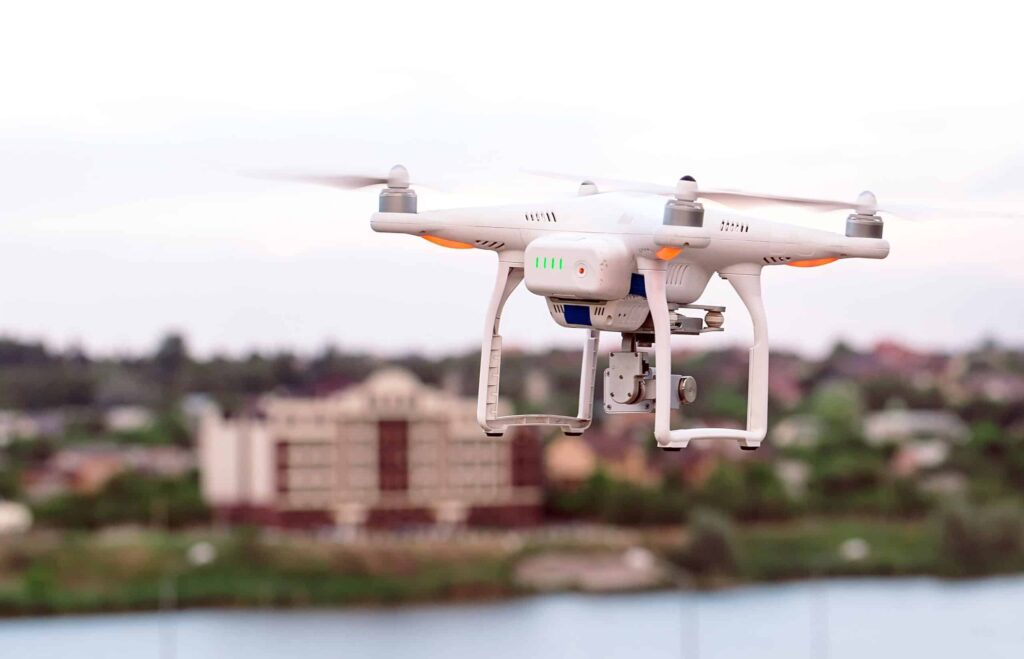
Introduction
The Security Technology Research and Innovation Grants (S-TRIG) Programme and the Department for Transport and Home Office’s Future Aviation Security Solutions (FASS) invited businesses and academic institutions to address future challenges within national security. Weisi Guo at Cranfield University took part in the programme from July 2020 to March 2021 where they submitted two projects, ARENA and the one described, Drone Classification for Airport Protection.
Company Bio
Cranfield University is one of the leading aerospace universities of the world, hosting both the UK’s Digital Aviation Research and Technology Centre (DARTeC), the Beyond Visual Line-of-Sight (BVLOS) drone corridor, and as well as its own airport and the UK’s national flying laboratory (host of the Queen’s Anniversary Prize).
Business Challenges
Drone data and classification is not understood very well, and training machine learning algorithms take a significant time to reach high accuracy. Cranfield University aim to improve the image classification accuracy of aerial drones by identifying the missing data needed for robust deep learning training.
A wide range of multi-spectral image and lidar equipment is currently being used to scan the airspace around national infrastructures, including airports and power stations. These often detect a range of objects on the horizon from bird flocks, to drones, to visual clutter. Correct detection and classification of drones, especially hostile ones, is critical to alerting the authorities and counter-drone systems to act. The current challenge is that most counter-drone detection systems have an accuracy of 89-94% outdoors in wild environments with very few drone samples, raising too many false positives and unknown performance metrics.
Cranfield University seek to improve the correct detection and classification for a wide range of camera and lidar equipment using the university as an ideal campus for testing drones. They aim to achieve a high accuracy (>99%) and low false positive rate, whilst covering a range of drone types and background / horizon clutter environments.
Collecting large amounts of drone data does not guarantee a full understanding of it and it is inefficient due to the diverse reconfigurable nature of drones and their proximity to ground clutter. Here, Cranfield University develop explainable deep learning for sparse data, where we sequentially acquire the minimum amount of missing data for training convergence, as opposed to just more data. In general, we expect this research to have foundational contributions to how industries facing sparse data learning challenges can still move forward in training robust machine learning algorithms.
Solutions
An S-TRIG grant award enabled the Cranfield University team to build a drone image database (4-5k data sets) that enabled deep learning algorithms to reliably detect drones (98-100% accuracy). They also developed a novel explainable AI method to continuously update the database with the most important missing images from a deep learning accuracy perspective.
To achieve this, the team trained generative adversarial networks (GAN) to identify the disparity between deep learning and true data feature distribution and used topological data analysis (TDA) to identify the missing data needed to complete training. This exposure allows identification of critical data that improves the learning accuracy and speed. Understanding how machine learning interprets drones can improve counter-drone ecosystems, especially against adversarial designs.
Cranfield University achieved 98-100% drone detection accuracy for high quality images. It was achieved by exposing what knowledge was missing in the AI using explainable AI, allowing reduction of false positive rates and updated the image data base from an airport protection perspective. This addressed the “Counter-Drones” airport protection challenge by directly demonstrating its success at Cranfield University research airport in a variety of scenario settings.
The project team developed the key technology to enable missing data detection and adversarial deep fake design. This allowed a wide range of stakeholders interested in detection and adversarial machine learning to improve their algorithms and combat against deep fake attacks. Cranfield University engaged several commercial stakeholders (see below) to explore joint test work and continued research to demonstrate impact through a newly awarded 2-year Royal Academy of Engineering Fellowship at Cranfield University on Drone Intention Prediction:
- Highways England (Chief Data Officer) and Department for Transport (Head of Counter-Drone Strategy) as candidates to engage with to ensure long term impact.
- Rinicom (CTO),
- Operation Solutions (Managing Director)
Benefits & Results
The S-TRIG grant funding enabled Cranfield University to progress drone classification technology for airport and other critical infrastructure protection. It enabled the University to showcase it to key organisations including Highways England and the DfT leading to a successful UKRI and Royal Academy of Engineering research grants.
Cranfield University secured funding for foundational research into AI, focused on adversarial machine learning for transportation. The £0.5M awarded by the Engineering and Physical Sciences Research Council (EPSRC) for project Mobility as a service: Managing Cybersecurity Risks across Consumers, Organisations and Sectors (MACRO), which started in June 2021, will enable continuation of the key foundational work.
Recently, Cranfield University were also awarded the 3.5 year £3m EPSRC/UKRI Trustworthy Autonomous Systems node in security. Since December 2020 it has worked with industrial partners including Thales, BAE Systems, Boeing, and HM Coastguard. Some of the funding will be used to enhance the foundation research, and to fund follow up experimentation.
Weisi’s team at Cranfield University have also been awarded a 2-year Royal Academy of Engineering Fellowship into Drone Intention Prediction as a direct result of this work. Department for Transport (DfT) have fed back on the interest and potential of this work in informing future Counter Drone strategies. There is interest from the Home Office, UK SMEs, and other departments which means further testing to build the necessary evidence base. Results have also been submitted to the Home Office JSARC C-UAS Industry Action Group.
“Drones represent an enormous number of applications that can make our world more efficient and safer but they also can create unknown threats. Weisi’s team at Cranfield are playing a key role in understanding and countering the potential and actual threats posed by drones. This small but important piece of work takes us a step closer to helping to identify and mitigate drone threats automatically to prevent disruption, loss of revenue and, potentially, life. The DfT and CPC also noted”Alex Weedon, Executive Director, SME Development and Academic Engagement
Counter drone is a strategic area of the Cranfield University and the international funding bodies. The main project outputs are:
(1) a continuously updated database of drones (currently 4000-5000 images made of real and synthetic images – See Figure 1),
(2) a deep learning classifier that can improve its accuracy (currently near 100% on sharp images) based on growing training data from database,
(3) a system that can identify what data is most missing from a deep learning knowledge perspective (see Figure 2), and
(4) adversarial drone designs that can fool detection systems. Whilst this was applied to drones, the general approach can be applied to a wide range of other machine learning areas.

