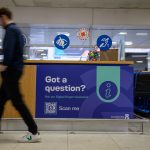
Intelligent Mobility – Research Papers
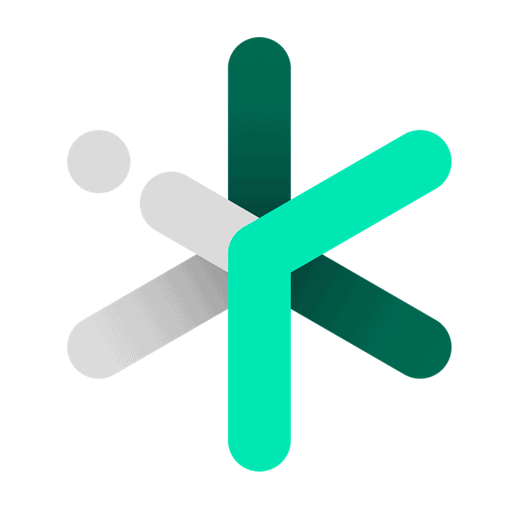
Explore Research Papers which have been produced from the Intelligent Mobility projects by our Capability team.
Regulating and Accelerating Development of Highly Automated and Autonomous Vehicles Through Simulation and Modelling
Autonomous vehicles (AVs) will be required to perform the driving task in the presence of a huge variety of road layouts, other road user movements and environmental conditions. This means the software and sensors that constitute the Automated Driving System (ADS) and enable a vehicle to operate in a highly automated or autonomous manner (e.g. SAE Level 31 and above), must be highly sophisticated, able to acquire appropriate contextual information and make safe and predictable decisions. Demonstration of confidence in such a system is a major step towards the introduction of AVs and intelligent mobility to our roads.
Specification Information to Inform Approvals for Advanced Vehicle Trials
The Centre for Connected and Autonomous Vehicles (CCAV) and the DfT’s International Vehicle Standards division (IVS) have instructed Connected Places Catapult (former Transport Systems Catapult) to deliver a report including a list of information (“the list”) that Government could ask an organisation (referred to in this report as “the testing organisation” or “AV developers”) seeking to apply for an exemption to a Construction and Use Regulation, to enable testing of automated vehicles on UK public roads in a way which is likely to be inconsistent with UK law, to help inform a decision by Ministers on whether to provide approval for that test. The list should be designed to elicit evidence to demonstrate that the test will be carried out safely and responsibly.
Ethical Considerations for Autonomous Vehicles
Autonomous systems (a category which includes AVs) have been proposed for use in multiple domains, with examples including nuclear containment, defence systems, health and transport. In this paper we discuss the ethical landscape surrounding the introduction and operation of autonomous vehicles as a form of transport on the public road network.
Use of autonomous vehicles in other domains (e.g. as a defence capability or a form of medical transport) is likely to impose ethical requirements which go beyond the scope of this document, and for which we refer the reader to existing literature.
Station Innovation 2 – Connected and Automated Vehicle Impact
This report summarises the output from Work Package 7 (titled ‘Autonomous and Connected Vehicles Impact’); a deliverable of the Station Innovation 2 project which has been undertaken by Connected Places Catapult (former Transport Systems Catapult) on behalf of the Department for Transport (DfT). This work package considers how Connected and Automated Vehicles (CAVs) may develop over the next decade and how these could be integrated with future station designs and operations in order to provide an efficient interchange in a multi-modal transport system.
Taxonomy of Scenarios for Automated Driving
This document has been prepared by the Connected Places Catapult (former Transport Systems Catapult) for the Department for Transport (DfT) and the Centre for Connected and Autonomous Vehicles (CCAV). This report represents the deliverable of the Project – ‘Taxonomy of Scenarios for Automated Driving’. Any views expressed in this report are not necessarily those of the DfT or CCAV.
Future Proofing Infrastructure for CAVs
This document has been prepared by Connected Places Catapult (former Transport Systems Catapult) for the Department for Transport (DfT) and the Centre for Connected and Autonomous Vehicles (CCAV). This report represents the deliverable of the Project – ‘Future Proofing Infrastructure for Connected and Automated Vehicles’. Any views expressed in this report are not necessarily those of the DfT or CCAV.
Short term traffic prediction on the UK motorway network using neural networks
The ability to predict traffic conditions over the short-term has the potential to improve traffic management by allowing decisions to be proactive to changing traffic conditions rather than reactive. This paper explores the application of a machine learning technique, specifically neural networks, to predict traffic conditions on a section of the UK motorway network 15 minutes ahead of time.